Navigating AI Governance, Risk, and Compliance (GRC): Key Risk Indicators (KRI) to Monitor
- aleccrawford6
- Oct 1, 2024
- 3 min read
Alec Crawford Founder & CEO of Artificial Intelligence Risk, Inc.
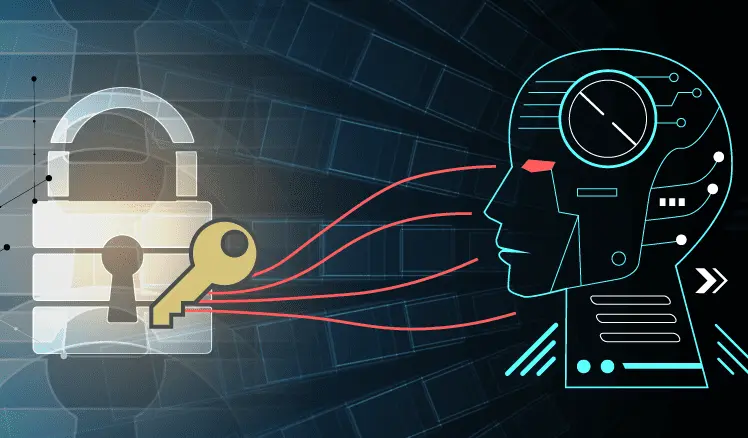
Artificial Intelligence (AI) is transforming industries with its immense potential, particularly through the deployment of Large Language Models (LLMs). However, with great power comes great responsibility, and that is where AI Governance, Risk, and Compliance (GRC) frameworks become crucial. This article identifies new Key Risk Indicators for AI GRC to facilitate safe and ethical AI deployment. We also believe that the world will soon extend beyond AI GRC to AI GRCC – including cybersecurity as the additional “C”.
Understanding AI GRC
AI GRC is an emerging framework that addresses the governance, risk management, and compliance needs specific to AI technologies. Many of these frameworks, such as the NIST AI RMF, are very general. The focus is on ensuring that AI systems are not only effective but also align with ethical standards and regulatory requirements. For businesses leveraging LLMs, AI GRC serves as a safeguard against legal, reputational, and operational risks. Here, we drill down to show very specific KRIs for AI GRC, many of them novel.
Key Risk Indicators (KRIs) in AI GRC
Bias -- yes, no, not applicable: Bias in data sets is inevitable. Testing for different types of bias with known data sets is important for LLMs. Nevertheless, the definition of “fairness” is not singular, and different models scoring fairness can give different results.
Explainability -- yes, no, not applicable. Explainability should only be tested for correct answers from the model, then if the model has a built-in explainability feature, it should be tested.
Privacy risk – yes, no. Personal identifying information is embedded in several pre–set queries and the percentage blocked or flagged by the system is the score. Higher is better from 0-100%.
Outlier identification (e.g. flagging that a question is for an area outside the model’s training) -- correct, false positive, false negative: Many AI models are not set up to detect outliers, nevertheless, if they are, it is important to measure accuracy with this KRI.
Robustness -- correct, incorrect, not related: Related to the prior KRI, robustness tests the model with prompts that are outside its training area. Humans are typically used to judge the answer.
Regulatory compliance – Compliance is related to the company’s industry, and a company may have multiple regulators. A data set can be constructed to test regulatory compliance from 0-100, with 100 being fully compliant. Likely, this will take multiple data sets and tests.
Stress testing -- correct answer, incorrect answer, unrelated answer, identification as outlier: This is the result for a question outside the model’s training. Note that some models can be set up to tell you that directly, hence that category of answer.
Prompt injection detection -- correct, false positive, false negative: Certain strings of characters can “jailbreak” a LLM and detecting them and blocking them, whether before sending to the LLM or within the model’s policy layer is important.
Do-anything-now (DAN style) attack detection -- correct, false positive, false negative: Certain commands or conversations can hijack an LLM and get it to say thing sit should not.
Blocked topic detection -- correct, false positive, false negative: If the topic is blocked (e.g. something illegal) in your system, blocking should be tested.
Implementing an Effective AI GRC Framework
To effectively manage these risks, organizations should develop a comprehensive AI GRC framework that includes the NIST AI RMF: Govern, Map, Measure, and Manage. The figure below shows how this aligns with AI LLM systems.

Conclusion
As AI continues to evolve, so do the risks associated with its deployment. By focusing on key risk indicators within an AI GRC framework, organizations can not only protect themselves from potential pitfalls but also harness AI's full potential responsibly and ethically. Monitoring and managing these indicators is an ongoing process, requiring vigilance and adaptability in a rapidly changing technological landscape. Embracing robust AI GRC practices ensures that AI advancements contribute positively to both business objectives and societal good. There is software that facilitates compliance with the NIST AI RMF today. Please see www.aicrisk.com for more information about AIR-GPT and the full white paper. It facilitates compliance with the NIST AI RMF as well as providing the world’s best user experience for CISOs to safeguard their AI.
Copyright © 2024 by Artificial Intelligence Risk, Inc. All rights reserved.
Comments